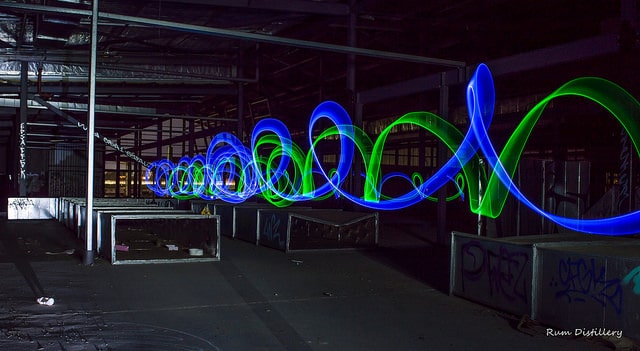
Chi square goodness of fit test specifically tells how well a categorical (nominal or ordinal) sample distribution fit into a hypothetical distribution. In other words, it is the test used to check if the sample data is consistent with a hypothesized distribution of the population. The goodness of fit test is used to determine the observed sample distribution matches or fits the expected values; hence we use the term goodness of fit.
The goodness of fit is a non-parametric test because it does not rely on estimates of a population parameter like mean or variance to make an inference on the characteristics of the population.
When to use Chi-Square Goodness of Fit?
A chi square goodness-of-fit test can be conducted when there is one categorical variable with more than two levels. If there are exactly two categories, then a one proportion z test may be conducted.
Reference.
The chi-square goodness-of-fit test requires 2 assumptions2,3:
1. Independent observations;
2. For 2 categories, each expected frequency EiEi must be at least 5.
For 3+ categories, each EiEi must be at least 1 and no more than 20% of all EiEi may be smaller than 5.
Reference
Structure of a Chi-Square Goodness of Fit Test
The goodness of fit tests is structured in cells; therefore, the observed frequency goes in each cell. Furthermore, the distribution you are trying to match would have a theoretical frequency. Then, the chi-square is summed across all cells.
Use the data values structured into cells, explicitly requiring a calculated chi-square test statistic. The unknown distribution is tested; likewise, the Degrees of Freedom vary according to the distribution.
GOF Distribution | Degrees of Freedom
Normal | # cells – 3
Poisson | # cells – 2
Binomial | # cells – 2
Uniform | # cells – 1
Steps to perform Chi-Square goodness of fit
Step1:
Firstly, define the null hypothesis and alternative hypothesis
- Null hypothesis (H0): There is no difference between the observed value and the expected value
- Alternative hypothesis (H1): There is a significant difference between the observed value and the expected value
Step 2:
Secondly, specify the level of significance.
Step 3:
Thirdly, compute the χ2 statistic.
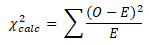
- O is the observed value
- E is the expected value
Step 4:
Fourthly, calculate the degree of freedom:
The degrees of freedom in chi-square test depends on the sample distribution

Step 5:
Then, find the critical value based on degrees of freedom.
Step 6:
Finally, draw the statistical conclusion:
If the test statistic value is greater than the critical value, reject the null hypothesis. Hence, we can conclude that there is a significant difference between the observed value and the expected value.
Chi-Square goodness of fit test Example 1: Did the Distribution Change?
Ten years ago, US airlines categorized the priority customers (those who completed 10,000 miles traveled in a year) based specifically on age:

Similarly, in 2020, 500 priority passengers were sampled, and below are the results:

At a 95% confidence level, would you conclude that the population distribution of priority customers changed in the last 10 years?
- Null hypothesis (H0): The sample data meet the expected distribution.
- Alternative hypothesis (H1): The sample data does not meet the expected distribution.
Level of significance: α=0.05:
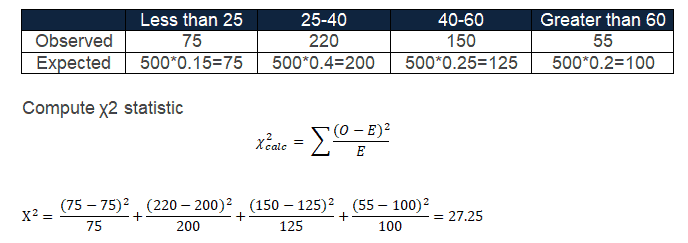
Degrees of freedom = number of categories (n)= 4
n-1 =3
Chi-square critical value for 3 degrees of freedom =7.815
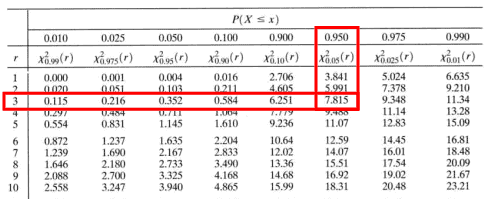
The test statistic value is greater than the critical value; hence, we can reject the null hypothesis.
So, we can conclude that the priority customers in 2020 are different than those expected based on the 2010 population.
Comments (4)
I think there is an error in the DOF calculation in the Chi Square Normality Test “Degrees of freedom = No of categories -3 =5-3 =2”.
DOF = (rows – 1) x (cols – 1)
DOF = (5-1) x (2 – 1)
DOF = 4 x 1
DOF = 4
Hello Greg Tilson,
If mean and standard deviation is not given, DF for chi-square normality test = No of categories -3
Thanks
If i may, What is the formula if mean and Std Dev are given?
Hello Ashwin,
df = number of intervals – 1, since the mean and standard deviation are given
Thanks