The Central Limit Theorem states that the distribution of the sample means approaches normal regardless of the shape of the parent population.
Sample means (s) will normally be more distributed around (µ) than the individual readings (Xs). As n– the sample size–increases, the sample averages (Xs means) will approach a normal distribution with mean (µ).
So, don’t worry if your samples are all over the place. The more sample sets you have, the sooner the averages of those sets will approach a normal distribution with a mean of (µ).
Significance of Central Limit Theorem
The Central Limit Theorem is important for inferential statistics because it allows us to safely assume that the sampling distribution of the mean will be normal in most cases. This means that we can take advantage of statistical techniques that assume a normal distribution.
The Central Limit Theorem is one of the most profound and useful results in all statistics and probability. The large samples (more than 30) from any sort of distribution of the sample means will follow a normal distribution.
The spread of the sample means is less (narrower) than the spread of the population you’re sampling from. So, it does not matter how the original population is skewed.
- The means of the sampling distribution of the mean is equal to the population mean µx̅ =µX
- The standard deviation of the sample means equals the standard deviation of the population divided by the square root of the sample size: σ(x̅) = σ(x) / √(n)
Assumptions
- Samples must be independent of each other
- Samples follow random sampling
- If the population is skewed or asymmetric, the sample should be large (for example, a minimum of 30 samples).
Why Central Limit Theorem is important
Central Limit Theorem allows the use of confidence intervals, hypothesis testing, DOE, regression analysis, and other analytical techniques. Many statistics have approximately normal distributions for large sample sizes, even when we are sampling from a distribution that is non-normal.
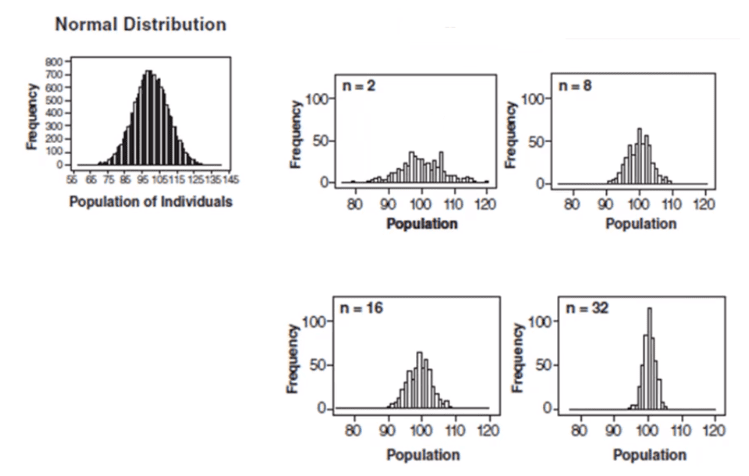
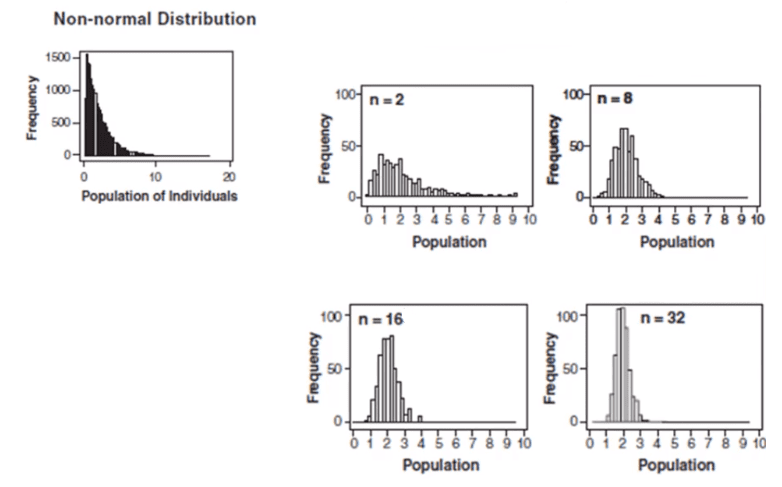
In the above graph, subgroup sizes of 2, 8, 16, and 32 were used in this analysis. We can see the impact of the subgrouping. In figure 2 (n=8), the histogram is not as wide and looks more “normally” distributed than Figure 1. Figure 3 shows the histogram for subgroup averages when n = 16, it is even more narrow and it looks more normally distributed. Figures 1 through 4 show, that as n increases, the distribution becomes narrower and more bell-shaped -just as the central limit theorem says. This means that we can often use well-developed statistical inference procedures and probability calculations that are based on a normal distribution, even if we are sampling from a population that is not normal, provided we have a large sample size.
Unimodal Distribution
Mode is one of the measures of central tendency. Mode is the value that appears most often in a set of data values or a frequent number. The Unimodal distribution will have only one peak or only one frequent value in the data set. In other words, Unimodal will have only one mode, the values increase first and reach to peak (i.e. the mode or the local maximum) and then decreases.
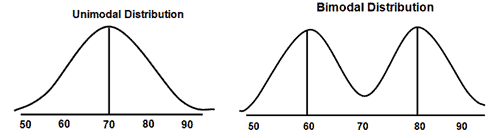
The normal distribution is the best example of a Unimodal distribution. Similarly, Bimodal distribution means there are two different modes, and multimodal means more than two different modes.
Central Limit Theorem Examples
Case 1: Less than
Example: A population of 65 years male patients with blood sugar was 100 mg/dL with a standard deviation of 15 mg/DL. If a sample of 4 patients’ data were drawn, what is the probability of their mean blood sugar being less than 120 mg/dL?
- µ = 100
- x̅ = 120
- n=4
- σ =15
Compute P(X<120): z= x̅- µ/ σ/√n = 120-100/15/√4=20/7.5=2.66
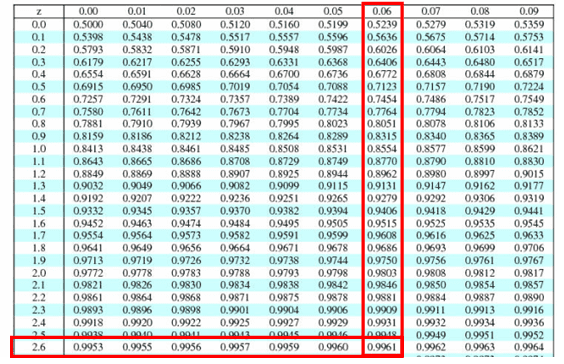
P(x<120) = z(2.66) = 0.9961 = 99.61%
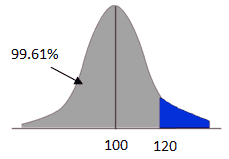
Hence, the probability of mean blood sugar is less than 120 mg/dL is 99.61%
Case 2: Between
Example: A population of 65 years male patients with blood sugar was 100 mg/dL with a standard deviation of 20 mg/DL. If a sample of 9 patients’ data were drawn, what is the probability of their mean blood sugar being between 85 and 105 mg/dL?
First, compute P(x<105)
- µ = 100
- x̅ = 105
- n=9
- σ =20
Compute the Z score z= x̅- µ/ σ/√n = 105-100/20/√9=5/6.67=0.75
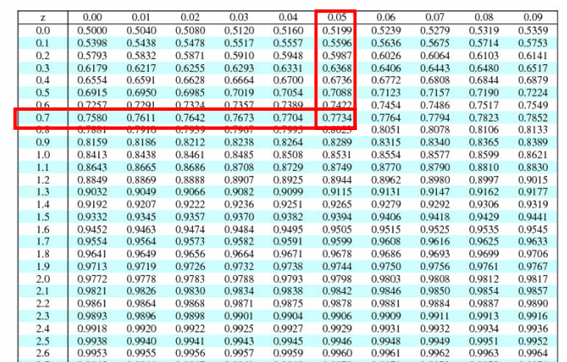
P(x<105) = z(0.75) = 0.7734 = 77.3%
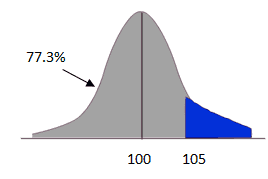
Then, compute P(x<85)
- µ = 100
- x̅ = 85
- n=9
- σ =20
Compute the Z score z= x̅- µ/ σ/√n = 85-100/20/√9=-15/6.67=-2.24
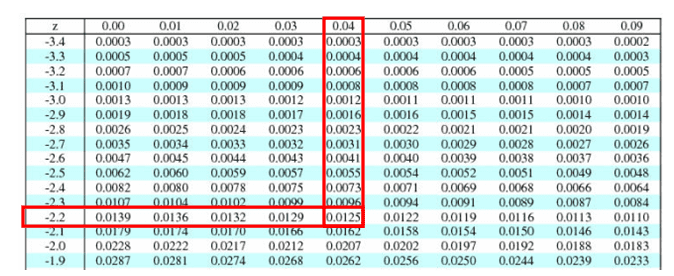
P(x<85) = z(-2.24) = 0.0125 = 1.25%
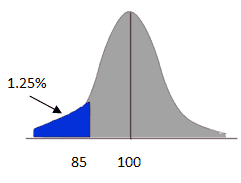
Since we are looking for blood sugar between 85 and 105 mg/dL P(85<x<105) = 77.3-1.25 = 76.05%
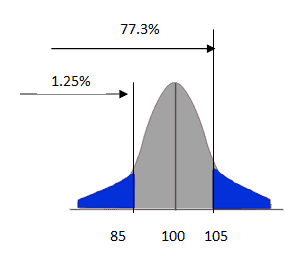
Hence, the probability of mean blood sugar is between 85 and 105 mg/dL is 76.05%
Case 3: Greater than
Example: A population of 65 years male patients with blood sugar was 100 mg/dL with a standard deviation of 20 mg/DL. If a sample of 16 patients’ data were drawn, what is the probability that their mean blood sugar is more than 90 mg/dL?
- µ = 100
- x̅ = 90
- n=16
- σ =20
Compute the Z score z= x̅- µ/ σ/√n = 90-100/20/√16=-10/5=-2
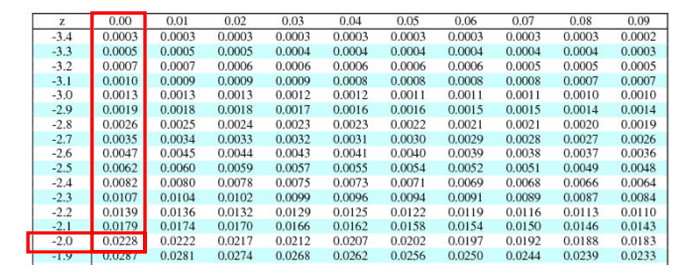
P(x<90) = z(-2.0) = 0.0228 = 2.28%
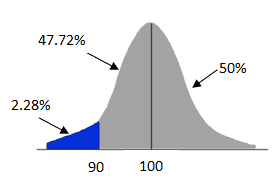
Since we are looking for blood sugar of more than 90 mg/dL =100%-2.28%=97.72%
Hence, the probability of mean blood sugar greater than 90 mg/dL is 97.72%
Jarque- Bera analysis.
Wikipedia has a good article here:
Comments (8)
Ted,
How did she get 34.13% on this problem ? can you please explain?
You should look up positive Z here a she states.
Remember, you’re looking for distance away from the center. Look at the chart you pasted in. See how the left tail is shaded? You want the opposite part shaded; the center to the limit.
Hi ,
I am really struggling with the video example (3rd video at approx. 1.45 min.), as it states that there are no negative Z scores and that we just look up a positive value in positive table. But there is a negative Z table and it gives you significantly different values, so the result is very different too. It is very confusing.
Am i right in thinking if you get a negative Z score you need to look it up in negative table – Left Tail Area?
Thank you
Maria
Hi Maria,
Thanks for the question. We normally only support the questions in our question bank with the reference pages and videos for help but I wanted to provide some clarity here.
That video is not very straight forward.
First, yes, there certainly can be negative z scores. A negative z-score reveals the raw score is below the mean average. For example, if a z-score is equal to -2, it is 2 standard deviations below the mean.
Second, in the video she’s showing that you can adapt a positive table (if that’s the only one you have). Notice that she comes up with that 90%+ figure then subtracts it from 100%? She’s taking advantage of the fact that the z scores are from a normal (and hence symmetric) distribution.
It would have been much more straight forward for her to have simply used the negative z table.
Hi Ted,
The standard deviation of the sample means equals the standard deviation of the population divided by the square root of the sample size: σ(x̅) = σ(x) / √(n)
This should be “s/sqrt(n)”, i.e. sample standard deviation as we don’t know population standard deviation.
This is also standard error, is my understanding correct?
Hi Lakshman,
Suppose we are sampling from a population with a finite mean and a finite standard-deviation(sigma). Then Mean and standard deviation of the sampling distribution of the sample mean can be given as:
µ(x̅)=µ and σ(x̅) = σ(x) / √(n).
Thanks
In Case 2: Between I seem to ‘Compute the Z score’ for more than 85 as -2.25 and not -2.24. Taking the same calculation of 85-100/20/√9
Hi Krishna Bholah,
The value of (85 – 100) / (20 / √9) is -2.24999. We can consider -2.25, but the value does not change much. The probability of mean blood sugar between 85 and 105 mg/dL is 76.05% for -2.24, whereas for -2.25, it is 76.08%.”
Thanks