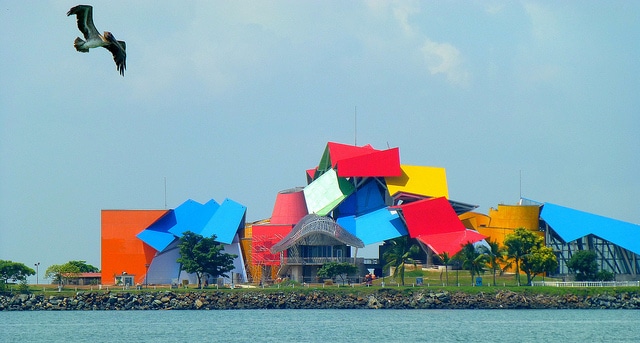
Because Full Factorial design experiments are often times cost-prohibitive when a number of treatment factors are involved, many people choose to use Partial/Fractional Factorial designs. These designs evaluate only a subset of the possible permutations of factors and levels. Generally, a Fractional Factorial design looks like a Full Factorial design for fewer factors, with extra factor columns added (but no extra rows).
Using Fractional Factorial design makes experiments cheaper and faster to run but can also create unclear interactions between factors.
For a good explanation of the reasoning behind using Fractional Factorial design, see Thomas B. Barker’s introductory video:
Fractional designs are typically only used for experiments with two-level factors, as designing experiments with more than two levels per factor can get difficult and messy quite fast.
Fractional Factorial Design Notation
The standard notation for Fractional Factorial designs is lk − p, where:
- l is the number of levels in each treatment factor.
- k is the number of treatment factors.
- p is the number of interactions that are confounded.
The fraction of trials required is calculated using this formula: 1/(lp).
For example, an experiment with two levels per treatment factor and two confounded interactions would require 1/(22) or 1/4 of the trials required for a Full Factorial design.
The notation used for the specific combination of factors being tested in a trial uses letters to designate a specific factor’s high (or second) level.
For example, in a three-factor experiment, we’d use a for the first factor, b for the second, and c for the third.
In a trial where a is at its high level and b and c are at its low levels, we’d use the notation a.
In a trial where both a and b are at their high levels and c is at their low level, we’d use the notation ab.
When creating a table of trial factors and levels, the low (or first) level is designated with a minus sign, and the high (or second) level is designated with a plus sign. This becomes important when we start generating the fractional design itself.
Generating the Fractional Design for an Experiment
When we create a Fractional Factorial design from a Full Factorial design, the first step is to decide on an alias structure.
Let’s look at a fairly simple experiment model with four factors. We know that to run a Full Factorial experiment, we’d need at least 2 x 2 x 2 x 2, or 16, trials. That’s too many, so we decided to confound one factor. That gives us values of:
l = 2
k = 4
p = 1
This experiment is classed as a 24-1 Fractional Factorial design.
If the experiment had only three factors, the (Full Factorial) design table would look like this:
Trial | Factor A | Factor B | Factor C | Notation |
---|---|---|---|---|
1 | – | – | – | (1) |
2 | + | – | – | a |
3 | – | + | – | b |
4 | + | + | – | ab |
5 | – | – | + | c |
6 | + | – | + | ac |
7 | – | + | + | bc |
8 | + | + | + | abc |
Our fractional 4-factor design uses the same table with the same number of trials but with an extra factor, D. To keep the number of trials the same, we’ll use a level of D in each trial that corresponds with the levels used in factor A and factor B, combined. This is where the plus and minus signs come in! We’ll use this simple formula:
D = A x B
So if A is at its low level (-) and B is at its high level (+), then (remembering back to grade-school math – a negative multiplied by a positive) D will be (-).
The experiment design table then looks like this:
Trial | Factor A | Factor B | Factor C | Factor D | Notation |
---|---|---|---|---|---|
1 | – | – | – | + | d |
2 | + | – | – | – | a |
3 | – | + | – | – | b |
4 | + | + | – | + | abd |
5 | – | – | + | + | cd |
6 | + | – | + | – | ac |
7 | – | + | + | – | bc |
8 | + | + | + | + | abcd |
If we had more treatment factors that we needed to account for, but we still wanted to keep the 8-trial model, we could introduce confounding along the lines of:
E = A x C
F = A x B x C
The Engineering Statistics Handbook has a handy summary table of Fractional Factorial designs.
Analyzing Fractional Factorial Designs
- Analyze Fractional Factorial designs using
- The average effect of factors
- Optimum score and performance
- The sum of squares scree plot
Why You Would Use Partial or Fractional Factorial Design Instead
One of the big drawbacks of Fractional Factorial design is the potential to miss important interactions.
Fractional Factorials (like Latin and Graeco-Latin Squares) will not allow an analysis of interactions. The interactions are confounded with other effects.
Helpful Partial and Fractional Factorial Video
Six Sigma Black Belt Certification Partial and Fractional Factorial Design of Experiment Questions:
Question: If the number of runs required for a Full-Factorial experiment is cost-prohibitive, which of the following experiments would have the same number of variables but fewer runs? (Taken from ASQ sample Black Belt exam.)
(A) Completely randomized factorial
(B) Replicated factorial
(C) Multilevel factorial
(D) Fractional factorial
Answer:
When you’re ready, there are a few ways I can help:
First, join 30,000+ other Six Sigma professionals by subscribing to my email newsletter. A short read every Monday to start your work week off correctly. Always free.
—
If you’re looking to pass your Six Sigma Green Belt or Black Belt exams, I’d recommend starting with my affordable study guide:
1)→ 🟢Pass Your Six Sigma Green Belt
2)→ ⚫Pass Your Six Sigma Black Belt
You’ve spent so much effort learning Lean Six Sigma. Why leave passing your certification exam up to chance? This comprehensive study guide offers 1,000+ exam-like questions for Green Belts (2,000+ for Black Belts) with full answer walkthroughs, access to instructors, detailed study material, and more.
Comments (2)
I believe that the sign structure for Factor D, from run order 1 through 4 is incorrect. It should go D=A*B*C. So signs should be – + + -. Thank you
Hi Felix,
If we have only 8 trials and we want to add an additional factor D, we cannot have a full factorial design because the number of unique combinations of factor levels would exceed the available number of trials.
However, we can still incorporate factor D into the design by using a fractional factorial design or a design that confounds factor D with other factors. This means that we would not be able to estimate the main effects and interactions involving factor D separately.
Author considered D= A*B, the above table is correct,
D=A*B
Trial Factor A Factor B Factor C Factor D Notation
—————————————————————-
1 – – – + d
2 + – – – a
3 – + – – b
4 + + – + abd
5 – – + + cd
6 + – + – ac
7 – + + – bc
8 + + + + abcd
Similarly, if you consider D= A*B*C, then
Trial Factor A Factor B Factor C Factor D Notation
—————————————————————-
1 – – – – (1)
2 + – – – a
3 – + – – b
4 + + – + ab
5 – – + – c
6 + – + – ac
7 – + + – bc
8 + + + + abc
Thanks