Confounding refers to a situation where the effects of two or more factors on a response variable cannot be separated or distinguished from each other. In other words, it represents a prevalent origin of bias and error in experimental design, impacting the validity and reliability of results and posing challenges in making causal inferences.
Confounding occurs when you can’t distinguish the effects of certain factor interactions because of other potential factor effects. This most commonly happens when:
- Uncontrolled or ‘nuisance’ factors are affecting the results.
- Partial factorial designs are used.
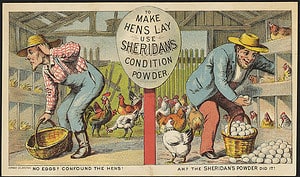
When Confounding occurs in DoE
Confounding may occur when uncontrolled variables are at play within the design, or when there is inadequate control over an independent variable.
A confounding variable is a factor that exhibits a relationship with both the independent variable and the dependent variable in an experiment. For instance, in a study evaluating the impact of a new drug on hair fall prevention, age could serve as a confounding variable because it influences both hair fall and the response to the drug. The presence of a confounding variable can lead to a misleading association between the independent and dependent variables, obscuring the genuine effect of the treatment.
Is Confounding a problem?
Confounding represents a distinct form of bias, impacting the accuracy of the association measure between an exposure and health outcome.
Confounding variables need to be considered to ensure the internal validity of the experiment. These confounding variables intertwine with a study’s independent and dependent variables. Emphasizing confounding variables becomes pivotal for ensuring the internal validity of the research. Failure to do so may introduce biases, rendering the results potentially misrepresentative of the actual relationships between variables.
How to identify the Confounding
Numerous techniques and instruments are available for identifying confounding in experimental data. Use graphical methods like scatter plots or box plots to visually assess the interplay between variables in the context of confounding. Additionally, statistical approaches like stratification and regression adjustment to manage confounding variables, allowing for the estimation of the treatment’s adjusted effect.
How to avoid or minimize the Confounding
To avoid confounding, it is crucial to meticulously plan the experiment. This involves clearly formulating research questions, determining the target population and sample size, specifying independent and dependent variables, and carefully selecting both the experimental design and the analysis methods.
However, it is not always possible to avoid it, to minimize the effect, researchers typically use techniques such as randomization and blocking. Randomization requires assigning experimental units randomly to different treatment groups to ensure an equal distribution of any confounding variables among the groups. Blocking involves grouping experimental units based on certain characteristics to reduce the impact of variables.
Example – A simple partial factorial design :
Trial | Factor A | Factor B | Factor C |
---|---|---|---|
1 | – | – | + |
2 | + | – | – |
3 | – | + | – |
4 | + | + | + |
In this experiment design, Fc = Fa * Fb (see Partial/Fractional Factorial Design if you need to revisit the notation used).
The experimental design is set up in a way that allows for the calculation of the interaction effect of Factor C (Fc) by multiplying the effects of Factors A (Fa) and B (Fb). The notation Fc = Fa * Fb signifies this relationship. Now, the key point here is that, based on this design, researchers cannot distinguish the effects of Factor C on the experiment from the interactions between Factor A and Factor B. This implies that the influence or impact of Factor C on the outcome is confounded with the combined effects of the interaction between Factor A and Factor B.
In other words, variations in the observed results cannot solely be attributed to Factor C, as its effects intertwine with the joint influence of Factors A and B interacting with each other. Researchers commonly encounter this experimental setup in partial or fractional factorial designs, where they select a subset of possible factor combinations to reduce the number of experimental runs while still capturing essential information about the factors and their interactions. This setup means that researchers cannot distinguish the effects of Factor C on the experiment from the interactions between Factor A and Factor B.
Example of Confounding
Example: A pharmaceutical company conducts a clinical trial to evaluate the effectiveness of a new drug in preventing hair fall (independent variable). The study aims to measure the reduction in hair fall as the primary outcome (dependent variable). Participants of varying ages are in the study.
Potential Confounding Variable:
The study design does not consider age, but age is related to both hair fall and the body’s response to the drug. Older individuals might experience natural hair thinning due to aging, and their response to the drug could differ from that of younger participants.
Confounding Effect:
If the researchers do not account for age, they might misinterpret the observed reduction in hair fall solely as the effect of the drug. For example, let’s say the overall reduction in hair fall in the study group is 30%. However, upon stratifying by age, researchers discovered that participants aged 50 and above experienced a 25% reduction, while those below 50 experienced a 35% reduction. Without considering age as a confounder, the study may not accurately attribute the observed effects to the new drug. In reality, age-related factors could influence the observed effects, and the drug’s impact may vary across different age groups.
Addressing Confounding:
To address confounding, researchers could analyze and report results stratified by age groups or use statistical methods to control for age as a potential confounder. For instance, accounting for age reveals that participants aged 50 and above experience a 25% reduction in hair fall, while those below 50 experience a 35% reduction. This nuanced analysis provides a more accurate interpretation of the drug’s impact on hair fall, considering age-related variations.
Block
Blocking can help limit its effect by distributing extra factors evenly across all experimental factors. In other words, Blocking in Design of Experiments (DOE) is a technique to control or account for known sources of variability that are not of primary interest in the study. By incorporating blocking, researchers aim to reduce the impact of these extraneous factors, making it easier to detect and estimate the effects of the primary factors of interest.
Comments (2)
Excellent and simple explanation about CONFOUNDING
Thank you! Glad it was helpful.