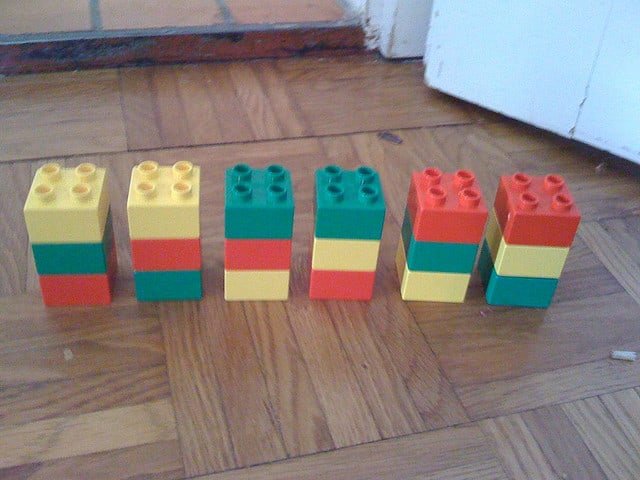
This Factorial Design Overview will cover one of the key issues in designing any experiment; identifying as many influences on the results as possible as well as either minimizing or isolating their impact on the results. These influences are accordingly known as factors.
Designing an experiment while taking all factors into account is known as factorial design.
Factorial Design Overview: Levels
There are two basic levels of factorial design:
- Full Factorial: includes at least one trial for each possible combination of factors and levels.
- Partial or fractional factorial: includes at least one trial for some, but not all, possible combinations of factors and levels.
Which one you choose depends on your particular experiment, the data being collected, and the budgetary/time constraints.
Each type of factorial experiment design has its pros and cons:
Pro | Con | |
Full factorial design | Thorough
High resolution | Expensive
Time-consuming Potential for too much data |
Partial factorial design | Cheaper
Faster | Low resolution
Could miss important interactions Allows confounding |
Factorial Design Overview Terminology
We’ve collated a list of the most common terms used in factorial design, along with their meanings, for easy lookup. See the factorial design terminology list.
Types of Factors
There are a number of different factors that could affect your experiments. We’ve listed the various types that you need to be aware of. Read more about factors.
Full Factorial Design
Full factorial design includes at least one trial for every combination of factors and levels. Read more about full factorial design.
Fractional Factorial Design
Fractional or partial factorial design includes at least one trial for a selection of factor-level combinations. Read more about fractional factorial design.
Confounding
Confounding can occur when factor interactions are not fully explored in an experiment’s design. Read more about confounding.
Blocking
Blocking is a method of minimizing confounding, where extra factors are distributed as evenly as possible across an experiment. Read more about blocking.
Comments (2)
Question #41: Latin square design was conducted on 9 acres of rice paddy field, P, Q& R denotes the different methods of cultivation, the experimental design and yield per acre are given below, calculate the sum of squares?
in case the full explanation for the math is in the link, this link is broken,
DOE Basics https://sixsigmastudyguide.com/design-of-experiments-2/
I am not sure why “3X3 Latin square design, for easy calculation, subtract 25 from each observation”
Cindy
Cindi – I’ve removed that link. The information is consolidated with the other 2 links in the write up.
I do agree that the question needs a better walkthrough. Will update asap.
Best, Ted.