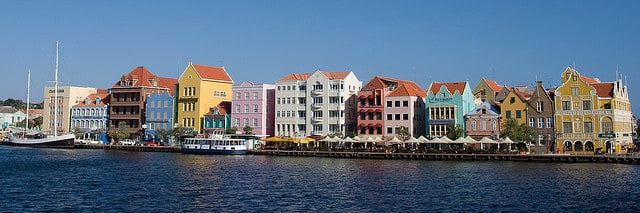
A p-value is used to decide whether hypothesis test results are statistically significant or not. Once it is calculated from analyzing test data, it is compared to the selected alpha level – if lower than the alpha level, the results are deemed to be statistically significant; if higher, the results are deemed to not be statistically significant.
A p-value is expressed as a number between 0 and 1.
This Khan Academy video further explains how they apply to hypothesis tests, in-depth:
While the p-value is a standard method for finding the key measurement of one’s results, there is a mix of opinions on whether it’s actually the ideal solution.
Geoff Cumming — Emeritus Professor at La Trobe University in Melbourne, Australia — explains his dislike of p-values in hypothesis testing in the following video:
But then, Jeff Leek and Rafa Irizarry do not agree, and their article lists a number of useful pieces of information about the p-value. To summarize (the full list, with explanations, is available in the linked article):
- They’re easy to calculate.
- They’re easy to understand.
- They have simple, universal properties.
- Their calibration is within useful error rates.
- They can be used in correlation with multiple tests.
- They’re reproducible.
Further Information About P-Values
What is the difference between an alpha level and a p-value? (PDF)
Comments (1)
Ciao! your work is extraordinary. Best 6 sigma training. In this page the pdf is no longer available. Can you add it again?
Massimo