When performing a hypothesis test, you are likely to encounter terms with which you may not be familiar. Unfortunately, this could hamper your ability to carry out hypothesis testing in a meaningful way. Likewise, you will find it hard to communicate your results to the stakeholders without the appropriate vocabulary. To avoid these issues, brushing up on your hypothesis-testing terminology is important. Let’s take a look at the most commonly used terms.
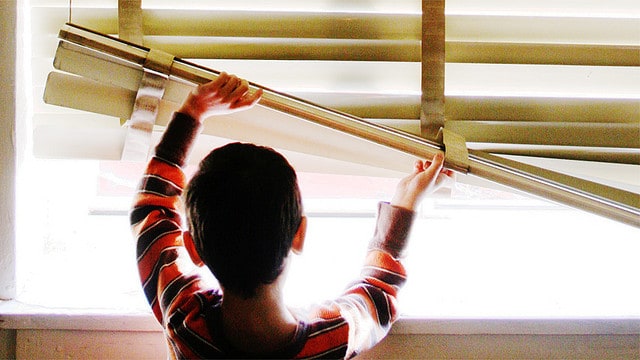
Alpha level
Also known as the alpha risk. The risk of committing a Type A error or incorrectly rejecting your null hypothesis is acceptable. An alpha level is always a number between 0 and 1—most commonly, people use a value of 0.05. Once your test is complete and you’ve run the data through statistical software, you’ll have a p-value to compare to your alpha level.
Alternative hypothesis
A hypothesis that disagrees with the null hypothesis; the two are mutually exclusive.
Beta level
Also known as the beta risk. It’s the acceptable risk of committing a Type B error–i.e., not rejecting your null hypothesis when it is, in fact, incorrect.
Conclusion
A statement that depicts the level of evidence (sufficient or insufficient), at what level of significance, and whether you reject (null) or support (alternative) the original claim.
Confidence level
Also known as the confidence interval. This refers to how confident you can be that your conclusion is, in fact, correct. The confidence level is easy to calculate: the alpha and confidence levels always add up to one. ie:
1 – α = confidence level
Critical region
Set of all values which would cause us to reject the null hypothesis H0. Also known as a rejection region.
Critical value(s)
The value(s) which separate the critical region from the non-critical region. The critical values are determined independently of the sample statistics.
A critical value separates the rejection region from the non-rejection region.
Decision
A statement based upon the null hypothesis. It is either “reject the null hypothesis” or “fail to reject the null hypothesis.” We will never accept the null hypothesis.
A p-value is the probability of getting a test statistic that is at least as extreme as the one found in the sample data.
Error
Two basic error types occur in hypothesis testing: type A errors, where you reject a correct hypothesis, and type B errors, where you accept an incorrect hypothesis. Read more about errors.
H0
Also known as the null hypothesis.
H1
Also known as the alternative hypothesis, or H(a).
Left-tailed test
The hypothesis test is left-tailed if the alternative hypothesis H1 contains the less-than inequality symbol (<).
Null hypothesis
The statement that you’re trying to disprove. Generally, this is the assumption that the experimental results are due to chance alone; nothing else influenced the results.
P-value
A p-value is a crucial element of any hypothesis test results. It’s a number between 0 and 1, and it gauges the probability that random fluctuations caused any data that might cause you to reject the null hypothesis. It’s calculated by running test results through a statistical significance test. If the p-value is lower than your alpha level, then you reject the null hypothesis. If higher, then you do not reject the null hypothesis. Read more about p-values.
Pooled (vs. Unpooled)
Pooling refers to the way in which the standard error is estimated when calculating terms for a hypothesis test.
The two proportions are averaged in the pooled version, and only one proportion is used to estimate the standard error. ASQ, Villanova, and most other organizations favor pooled calculations.
In the unpooled version, the two proportions are used separately. IASSC generally favors unpooled.
(Source)
Here’s a good read on when to use which in practice.
Rejection region
Also known as a critical region.
Right-tailed test
If the alternative hypothesis H1 contains the greater-than-inequality symbol (>), the hypothesis test is right-tailed.
- In hypothesis testing, when performing a right-tailed test, we reject the null hypothesis if the test statistic is larger than the critical value.
- Only when the test statistic is larger than the critical value will we be able to reject the null? Usually (emphasis on “usually”), we hope to reject the null because that means that our efforts are not in vain. If you are testing the null hypothesis and you are hoping that you have not adversely affected the process, you would then be hoping NOT to reject the null.
Significance level
Also known as the alpha level.
The probability of rejecting the null hypothesis when it is true: alpha = 0.05 and alpha = 0.01 are common. If no level of significance is given, use alpha = 0.05. The level of significance is the complement of the level of confidence in estimation.
The significance level (denoted by Alpha) is the probability that the test statistic will fall in the critical region when the null hypothesis is actually true.
Test statistic
A sample statistic is used to decide whether to reject or fail to reject the null hypothesis.
Two-Tailed Test
A two-tailed test is one with two rejection regions. If the null hypothesis has an equal sign, then this is a two-tailed test, and you can use the test statistic to reject the null hypothesis if the test statistic is too large or too small.
H0: µnew = µcurrent
Ha: µnew ≠ µcurrent
H0: µnew = µcurrent Ha: µnew is not = µcurrent
For example, if the null hypothesis has an equal sign, then this is a two-tailed test, and you can use the test statistic to reject the null hypothesis if the test statistic is too large or too small.
Comments (7)
Good stuff. Thanks allot.
Glad it helps, Chan!
Do we have to memorize all the formulas for the exam?
Hi Jeremy,
This depends on the organization you’re preparing for.
ASQ is open book. So, for organizations like that you don’t have to memorize, but you do have to collect all of the possible reference material you might want ahead of time.
IASSC is NOT open book. But they provide a reference sheet and you’re not allowed anything else. You can request a copy of the reference sheet from them.
Best, Ted
Hi, what would be the probability of the test statistic falling in the critical region, whether the null hypothesis is true or false?
Sam, are you referring to the significance level (alpha)?
H0 or Null hypothesis, from my understanding, I assumed it represented the true value or outcome that we hypothesize that we are trying to prove or disprove with experimentation. But from your definition it seems almost like the opposite, and that you are trying to disprove this hypothesis.
My idea of a Null hypothesis would be hypothesizing that combining a combination of metal 1 and metal 2 to make a compound metal would increase tensile strength, and it achieving that result. Therefore the Null Hypothesis was proven correct.
Is this understanding correct? Could you please help give me some clarification or an example of one?